刘鸿斌,李祥宇,杨 冲.基于PCA降维模型的造纸废水处理过程软测量建模[J].中国造纸学报,2018,33(4):50-57 |
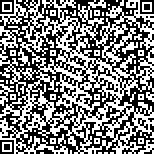 二维码(扫一下试试看!) |
基于PCA降维模型的造纸废水处理过程软测量建模 |
Soft Sensor Modeling of Papermaking Waste Water Treatment Process Using PCA Dimensional Reduction Models |
|
DOI:10.11981/j.issn.1000-6842.2018.04.50 |
中文关键词: 造纸废水处理 主成分分析 支持向量回归 人工神经网络 偏最小二乘 软测量 |
Key Words:papermaking waste water treatment processes principal component analysis support vector regression artificial neural network partial least squares soft sensor |
基金项目:制浆造纸工程国家重点实验室开放基金资助项目(201813,201610);南京林业大学高层次人才科研启动基金(163105996);江苏省制浆造纸科学与技术重点实验室开放基金项目(201530)。 |
|
摘要点击次数: 4510 |
全文下载次数: 1186 |
中文摘要: |
针对造纸废水处理过程的复杂特性,本课题将主成分分析(PCA)与人工神经网络(ANN)和支持向量回归(SVR)相结合,构建出两种新的软测量模型:主成分分析-人工神经网络(PCA-ANN)和主成分分析-支持向量回归(PCA-SVR)。本课题将这两种软测量模型应用于造纸废水处理过程中出水化学需氧量(COD)和出水悬浮固形物(SS)浓度的预测。计算结果表明,PCA-ANN和PCA-SVR的预测效果均优于偏最小二乘、支持向量回归和人工神经网络3种常规软测量模型,并且PCA-ANN的预测效果最优。对于出水COD浓度预测,PCA-ANN的决定系数(R2)为0.984,均方误差(MSE)为1.892,较ANN分别优化了9.7%和71.5%。对于出水SS浓度预测,PCA-ANN的R2为0.762, MSE为0.228,较ANN分别优化了31.2%和58.7%。 |
Abstract: |
To deal with the complicated characteristics of papermaking waste water treatment process, two new soft sensors based on dimensional reduction models, where principal component analysis (PCA) was combined with support vector regression (SVR) and artificial neural network (ANN), respectively, were developed for the prediction of the effluent chemical oxygen demand (COD) concentration and the effluent suspended solids (SS) concentration in a papermaking waste water treatment process. Conventional modeling methods including partial least squares (PLS), SVR, and ANN were used for comparison purpose. The results showed that the prediction performance of the PCA-based dimensional reduction models was better than that of the conventional models. Furthermore, PCA-based ANN (PCA-ANN) showed the most accurate prediction results. In terms of the prediction of the effluent COD concentration, the coefficient of determination (R2) and mean square error (MSE) of PCA-ANN were 0.984 and 1.892, respectively, which were optimized by 9.7% and 71.5% in comparison with ANN respectively. Compared with the prediction results of the effluent SS concentration based on ANN, the R2 (0.762) of PCA-ANN was increased by 31.2% and the MSE (0.228) of PCA-ANN was decreased by 58.7%. |
查看全文 查看/发表评论 下载PDF阅读器 HTML |