刘鸿斌,宋 留.相关向量机对废水处理系统出水水质的预测[J].中国造纸学报,2019,34(2):53-59 |
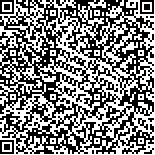 二维码(扫一下试试看!) |
相关向量机对废水处理系统出水水质的预测 |
Prediction of Effluent Quality in Wastewater Treatment Systems Using Relevance Vector Machine |
|
DOI: |
中文关键词: 相关向量机 降维方法 支持向量机 造纸废水处理 软测量 |
Key Words:relevance vector machine dimensionality reduction support vector machine papermaking wastewater treatment process soft sensor |
基金项目:制浆造纸工程国家重点实验室开放基金资助项目(201813);南京林业大学高层次人才科研启动基金(GXL029)。 |
|
摘要点击次数: 3864 |
全文下载次数: 1151 |
中文摘要: |
准确预测出水水质对造纸废水处理过程具有重要意义,为此笔者提出一种基于相关向量机(RVM)的软测量模型。首先,利用偏最小二乘法(PLS)提取实际造纸废水处理过程数据的潜变量,解决过程变量的共线性和高维度问题,然后利用潜变量建立RVM预测模型。结果表明,与RVM模型相比,本文提出的PLS-RVM组合模型在对出水悬浮固形物(SS)的水质预测测试时,均方根误差降低了7.76%,决定系数提高了12.32%;但对出水化学需氧量(COD)的预测测试效果提升并不明显。此外,PLS-RVM模型的预测效果较PLS-LSSVM模型有显著提升:对出水SS的预测,均方根误差降低了9.16%,决定系数提高了15.29%;对出水COD的预测结果中,均方根误差降低了9.29%,决定系数提高了18.34%。 |
Abstract: |
Predicting effluent quality precisely is important for papermaking wastewater treatment processes. In this paper, a soft sensor model based on relevance vector machine (RVM) was proposed to predict the effluent quality in a papermaking wastewater treatment plant. The proposed method included two steps. In the first step, partial least squares (PLS) method was used to extract the latent variables of the papermaking process data, which could solve the problems of collinearity and high dimensionality in the process variables. A RVM model using the extracted latent variables was developed in the second step. The results showed that the prediction performance of the PLS-RVM was better than that of the RVM for the prediction of the effluent quality in a papermaking wastewater treatment plant. With regard to the prediction of effluent suspension solids (SS), the root mean square error (RMSE) was decreased by 7.76% and the determination coefficient (R2) was increased by 12.32%; however, the prediction results were not improved significantly for the prediction of effluent chemical oxygen demand (COD). In addition, the prediction performance of the PLS-RVM was better than that of the PLS-LSSVM model for both the effluent SS and the effluent COD. In terms of the prediction of the effluent SS, the RMSE was decreased by 9.16% and the R2 was increased by 15.29%. In terms of the prediction of the effluent COD, the RMSE was decreased by 9.29% and the R2 was increased by 18.34%. |
查看全文 查看/发表评论 下载PDF阅读器 HTML |