高乐乐,周 强,王伟刚.基于CNN的深层次特征提取纸病辨识方法研究[J].中国造纸学报,2019,34(4):52-58 |
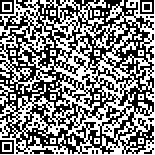 二维码(扫一下试试看!) |
基于CNN的深层次特征提取纸病辨识方法研究 |
Paper Defects Recognition Based on Deep Layer Feature Extraction of CNN |
|
DOI:10.11981/j.issn.1000-6842.2019.04.52 |
中文关键词: 纸病检测 难点纸病 卷积神经网络 深层次特征 分类器 |
Key Words:paper defect detection difficult paper defect convolution neural network deep feature classifier |
基金项目:陕西省教育厅专项科技项目(16JK1105);陕西省科技攻关项目(2016GY-005);咸阳市科技计划项目(2017K02-06)。 |
|
摘要点击次数: 4351 |
全文下载次数: 0 |
中文摘要: |
针对当前纸病检测方法中纸病特征量难分离以及难点纸病特征量难构建的瓶颈问题,提出基于卷积神经网络(CNN)的纸病辨识方法。该方法根据纸病图像的特点设计了纸病检测的CNN结构,在此基础上利用CNN自动提取纸病图像的深层次特征,结合Softmax实现对纸病的辨识。实验结果表明,基于CNN的深层次特征提取纸病辨识方法优于现有的纸病检测方法,能够对包括难点纸病在内的各种纸病进行精确辨识。 |
Abstract: |
To solve the bottleneck problems of difficult separation of paper defect feature quantities and difficult construction of the difficult paper defect feature quantities in current paper defect detection methods, a paper defect identification method based on convolution neural network (CNN) was proposed. First of all, according to the characteristics of paper defect image, the CNN network structure of paper defect detection was designed. On this basis, the deep features of paper defect image were extracted automatically by CNN, and the recognition of paper defect was realized by combining with Softmax. The experimental results showed that this method was superior to the existing detection methods, and was able to accurately identify all kinds of paper defect, including difficult paper defect. |
查看全文 查看/发表评论 下载PDF阅读器 HTML |